Exploring Machine Learning to Map Yellow Fever Risk
PAHO/WHO and UNICEF join forces to develop innovative methods for infectious disease risk mapping in the Americas
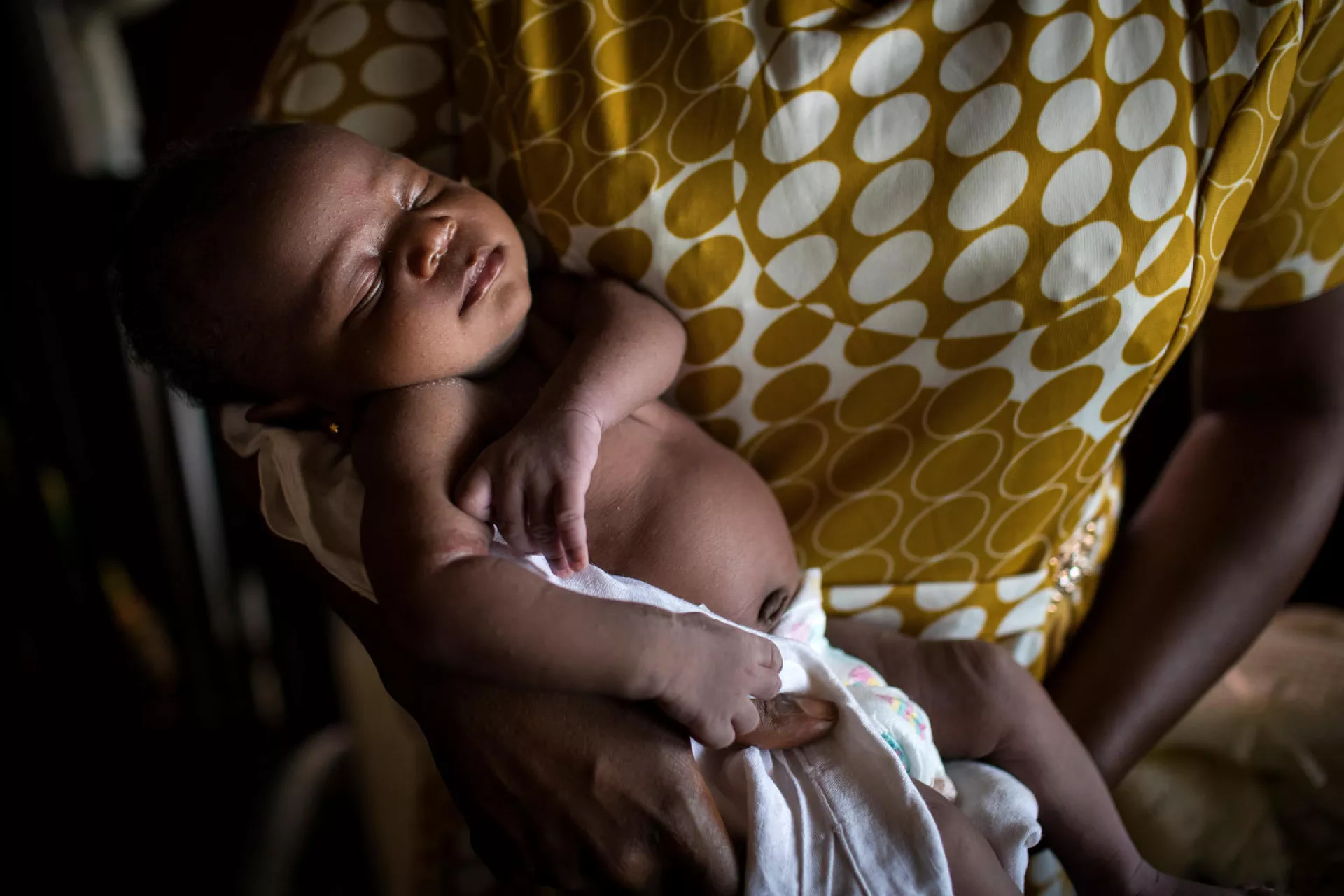
Authors
UNICEF: Elisa Omodei , Ivan Dotu, Manuel Garcia-Herranz
PAHO: Sylvain Aldighieri, Enrique Perez, Cristina Schneider, Patricia Nájera Hamrick, Jisoo Kim, Tshewang Dorji, Ana Rivière Cinnamond
Yellow fever is an endemic disease in Africa and the Americas. Periodically, sylvatic transmission via Haemagogus or Sabethes vectors have caused outbreaks of varying magnitude and extent in tropical areas of Brazil. However, beginning in December of 2016, Brazil suffered a massive epidemic that affected coastal areas where yellow fever had not been registered for decades. Between July 2016 and June 2018, over 700 people died from the hemorrhagic viral disease during the historic outbreak that recorded over 2,100 cases, triggering massive vaccination campaigns and threatening the vaccine stockpile.
Characterizing the rapid and expansive spread of yellow fever to new geographic areas became an important mission for PAHO. Yellow fever transmission in the Americas is closely tied to environmental factors due to the predominant sylvatic transmission pattern. This means the disease is transmitted to humans via mosquito vectors in tropical forested environments where virus persists between monkeys and tree-dwelling mosquitos. Environmental factors such as rainfall, habitat, altitude, temperature and presence of monkey and mosquito species make up conducive drivers for viral circulation. Yellow fever risk assessment using these factors is key to designing vaccination and other intervention campaigns aimed at reducing the burden of the disease.
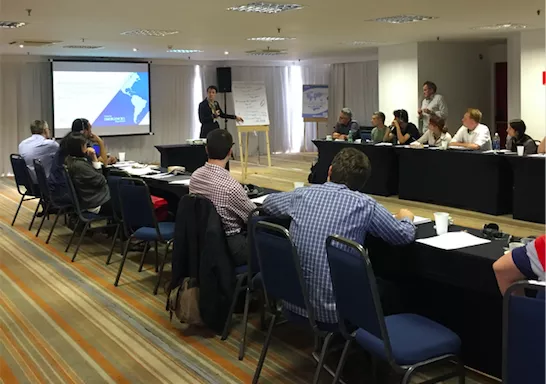
Last year, PAHO’s Health Emergencies Department and UNICEF’s Office of Innovation joined forces to explore the potential of machine learning to predict areas of yellow fever incidence in the Americas and assess the importance of geographic and environmental factors, employing PAHO’s seminal work and unique datasets. Increasing availability of digital data and development of Machine Learning (ML) techniques, and Artificial Intelligence (AI) in general, has proven extremely useful in understanding patterns of disease and health dynamics in populations. This trend of popular field of research called digital epidemiology uses digital data collected and generated inside and outside the public health system.
Using the machine learning algorithm AdaBoost and considering features like precipitation, temperature and primate prevalence, we were able to recall 100% of the cases reported in the Americas between 2000 and 2018 with 95% precision. That is, the 640 municipalities that have had yellow fever cases were all correctly recalled, and 31 yellow fever free counties were predicted to have cases. Most of these 31 counties border with infected counties, as shown in the figure below, making them suitable candidates for the appearance of new cases. Moreover, results showed that the most relevant features for risk prediction are latitude, canopy loss (% of the county with tree canopy loss >30%), ecoregion classification, number of nonhuman primates, and temperature. The full report can be downloaded here.
The work was presented in November 2018 at a workshop conveyed by PAHO in Brasilia on “Data Modeling Applied to Yellow Fever”, which brought together PAHO, UNICEF, the Brazilian Ministry of Health, as well as researchers from Imperial College London, University of Minnesota, Fundação Oswaldo Cruz (Fiocruz), and the University of Surrey. The workshop facilitated a dialogue between policy makers at the Brazilian Ministry of Health and the modelling community to address the uses of this type of research in yellow fever preparedness and outbreak response.
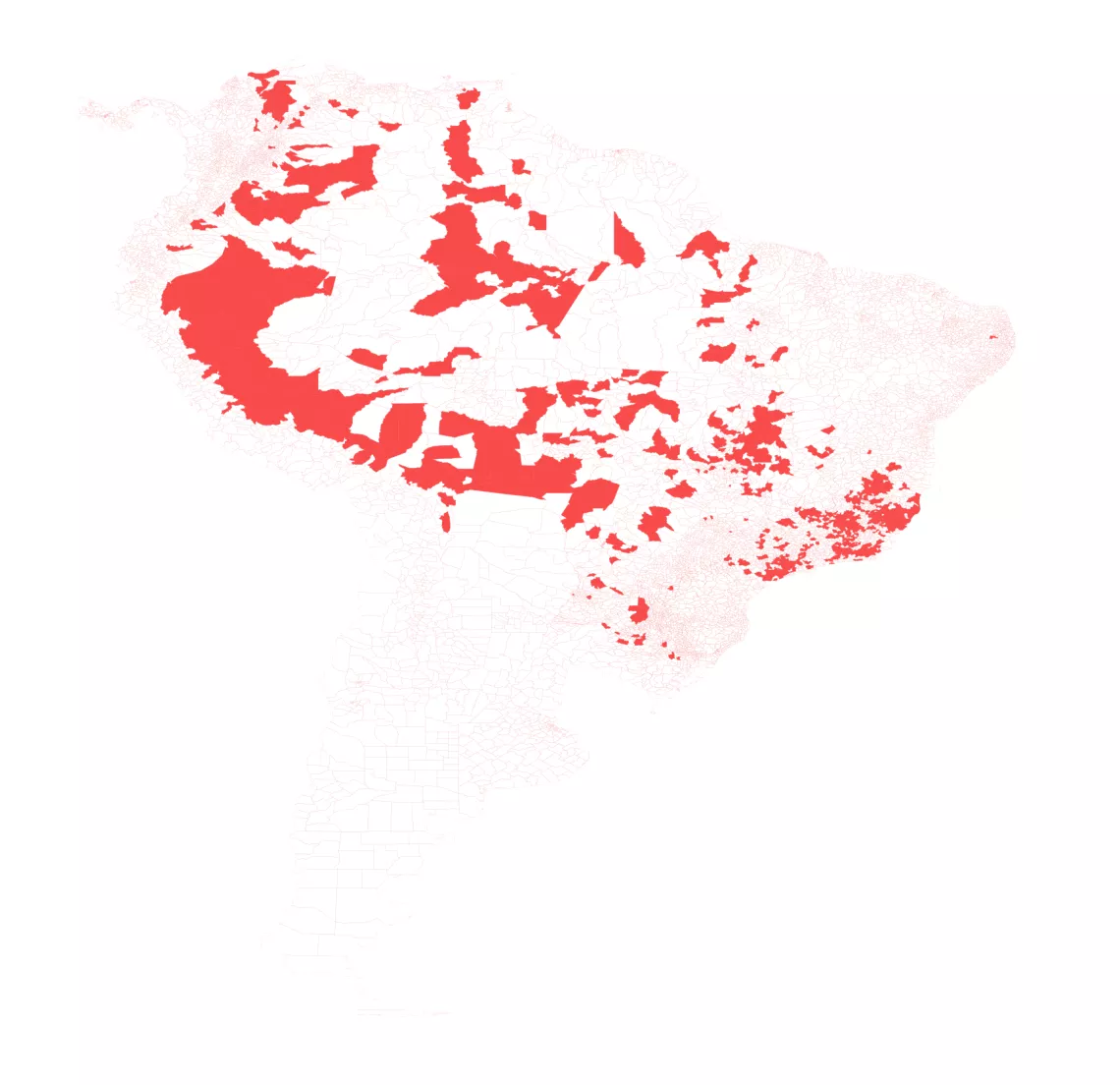


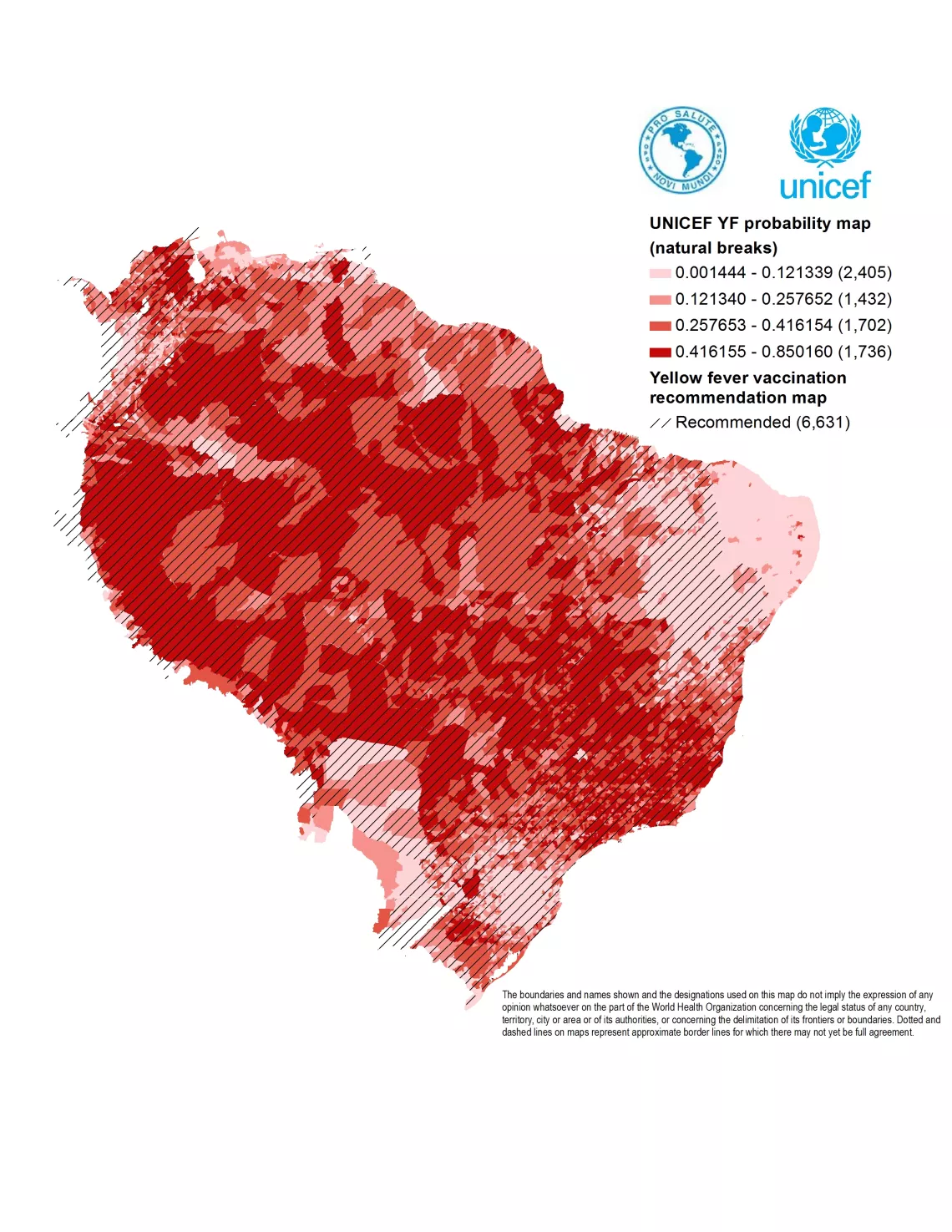
By identifying relevant environmental drivers that are conducive for yellow fever circulation, we can model likely scenarios and areas where the disease might be detected. By exploring machine learning techniques to estimate epidemic risk we open the door to faster and cheaper mapping, and to the incorporation of new data sources like satellite imagery or social media, too noisy and too big to be analyzed with classic statistical techniques. These innovative types of analysis will add to the knowledge of yellow fever epidemiology and have implications in preparedness and response for the next outbreak, not only in Brazil but any country at risk as per the yellow fever vaccinations for South America the probability map produced with ML technique vastly overlaps with the yellow fever recommendations map valid up to 2018. In 2019, Brazil continues to report cases, along with Bolivia and Peru, among 13 countries in the Region of the Americas where conditions are favorable for yellow fever transmission. Our continued work hopes to identify known and unknown areas with high risk of disease to implement appropriate, timely, and cost-effective preparedness and controls to reduce yellow fever spread and severity.